[China Pharmaceutical Network Technology News] Recently, Pennsylvania's Geisinger Health System and New York's Regeneration Pharmaceutical Co., Ltd. plan to acquire 250,000 people's genome sequencing data; in 2014, the UK launched a 100,000 genome project The United States and China have also announced plans for a million-person genomic data. It is understood that in order to promote the clinical application of genomic research, large-scale research projects at various national levels have been launched, and more and more hospitals and service providers around the world have begun genome sequencing of patients with cancer or rare genetic diseases. .
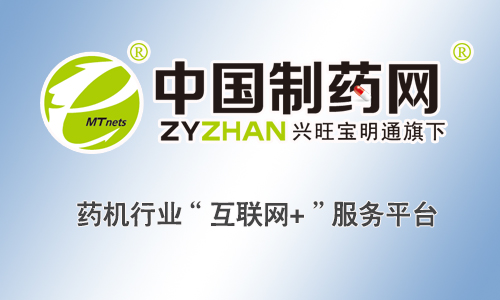
Seven major problems in the application of gene big data to clinical application need to be overcome
Massive data will bring unprecedented pressure on computational analysis and storage. It is estimated that genomics will soon exceed YouTube's data volume. But many researchers believe that today's big data is not rich enough and has no clinical value. "I don't know if 1 million is enough, but obviously we need more," said Marc Williams, dean of the Gesinger Genomics Medical Institute.
Application of variant data to clinical challenges and practices
Single nucleotide level mutation
At present, many research institutions mainly use exome sequencing, which can reduce the data analysis workload by nearly 100 times compared with the whole genome. However, there are still more than about 13,000 single nucleotide mutations identified. About 2% of these affect protein translation, and finding the disease-causing mutations is a daunting challenge.
For decades, researchers have put the single nucleotide mutation information they found into public resource databases, such as the dbSNP database. However, these mutational information often comes from the cellular level, animal models and even theoretical predictions, and is not sufficient for clinical diagnosis. In many cases, the level of evidence that these mutations are associated with the disease is very low.
Structural variation
Repeated or deleted genomic sequences that make clinical application more complicated. Existing sequencing techniques are difficult to detect structural variations. There are millions of variations between individuals within the genome-wide scope. Many of these mutations are located in non-coding regions, which do not encode proteins but have the effect of regulating gene activity and are still pathogenic. Since the scope and function of the non-coding region are difficult to define, even if the variation information can be obtained, clinical interpretation cannot be performed in a short period of time.
In response to these problems, people are working hard to solve them. For example, the National Human Genome Research Institute has established a Clinical Genome Resource Library, a disease-related mutation database with mutation information and supporting evidence to guide medical treatment. GenomicsEngland is advancing this by establishing a “clinical interpretation partnership†where doctors and researchers collaborate to build powerful disease-genotype association models.
The demand for large queues is obvious
Some "malignant" mutations are usually eliminated during evolution and are often rare, requiring large sample sizes for testing. Therefore, the establishment of a statistically significant model of mutation and disease-related correlation also requires a large number of patients.
Iceland's deCODE Genetics combines 150,000 genomic data (including 15,000 genome-wide sequences) with genealogy and medical history to infer the distribution of known genetic risk factors among the population, including breast cancer, diabetes and Alz Mutant mutations associated with the disease. As the company's CEO, Kári Stefánsson, said, "We have built 10,000 Icelandic databases with functional deletion mutations. We are investing a lot of energy to figure out how these gene deletions affect individuals."
The success of deCODE Genetics in this work is due to the genotype homogeneity of the Icelandic population, however, a broader genetic profile is required for Other projects. For example, the International Thousand Genome Project has catalogued some genetic diversity data, but most of the data are heavily biased towards Caucasians, making these data less useful for clinical research.
In addition, some of the questions are also derived from the reference genome. The first reference genome version is a patchwork of random breeder genes from several different races, but the latest version, GRCh38, integrates more information about the diversity of the human genome.
Generic data talent and computing power
Genomic or exome sequencing of large populations produces up to 40 PB (40 million GB) of data per year. In contrast, raw data storage is not the primary problem, and the bigger problem is the analysis of massive amounts of mutation data. Marylyn Ritchie, a genomics researcher at Penn State University, said, "The amount of computation is linear with the number of people. As variables and combinations increase, the amount of computation increases exponentially." If the increased data correlates with clinical symptoms or gene expression, Then the analysis will become more difficult. The processing of huge amounts of data from thousands of people may jeopardize many of the current statistical analysis tools. Ritchie said, "In the fields of meteorology, finance and astronomy, I have been exploring different types of data for a long time. I have communicated with people from Google and Facebook. Although our big data is different from them, we We should communicate more and apply their experience to our field."
However, unfortunately, many excellent programmers with big data mining experience have been attracted to Silicon Valley. Philip Bourne, deputy director of data science at the National Institutes of Health, believes that the scientific evaluation system is not suitable for these talents. Although some of these people really want to be scholars in the field of gene big data, they do not get a scholarship.
In addition to talent, data processing capabilities are another limiting factor. Gene big data often requires massively parallel computing using hundreds or thousands of large CPUs. To this end, many teams are turning to the "cloud" to store and analyze large amounts of data. TimHubbard, director of bioinformatics at Genomics England, said, "People are gradually getting this idea: applying algorithms to data." GenomicsEngland's cloud computing relies on government facilities, and its external access is strictly controlled. For other research institutions, the analysis of gene big data has gradually turned to commercial cloud systems, such as Amazon, Google and Ali's cloud services.
How to achieve data sharing and collaboration
In principle, cloud-based hosting encourages sharing and collaboration between databases. But highly sensitive clinical information and patient consent and privacy involve difficult ethical and legal issues.
In the European Union, different data processing rules between Member States hinder cooperation. Sharing data with non-EU countries relies on cumbersome mechanisms to establish data protection, and sharing data with private organizations requires a restrictive bilateral agreement. To help solve this problem, the Global Alliance for Genomics and Health has developed the Framework for Responsible Sharing of Genomic and Health-Related Data. The framework includes guidelines for privacy and informed consent, as well as the responsibilities and legal consequences of the organization that violates the rules.
Bartha Knoppers, bioethicist at McGill University in Canada and chairman of the Alliance's Regulatory and Ethical Working Group, said, “When signing a data transfer agreement, if the signatories agree to abide by the framework, they save a lot of work.†The framework allows Research organizations analyze shared genomic data while protecting privacy. Knoppers explained, “We hope to link these data to clinical data and medical records in the context of masking patients, otherwise we will not be able to achieve precision medicine.â€
In addition, the inclusion of genomics information in electronic medical archives has become increasingly important in many European countries. Hubbard said, "Our goal is to integrate it into the standard universal health insurance system." The UK's "100,000 genomics program" is at the forefront of this, but other countries are close behind. For example, Belgium recently announced a plan to explore medical genomics.
All of these countries benefit from this government-led public health insurance system. In the United States, the situation is more complicated. In addition to the public health insurance system, Medicare and Medicaid, the private health insurance system in the United States is very developed. Different medical insurance companies use different medical file systems, which makes the genome The integration of data has become difficult. In 2007, the National Institutes of Health funded the establishment of an electronic medical record and genomics network system (eMERGE) for big data integration and systems management analysis.
Clinical pharmacogenomics: from data to diagnosis
The integration of genomic data in medical records is mainly for doctors to provide reference for the diagnosis and treatment of diseases, one of which is pharmacogenomics. The Clinical Pharmacogenomics Implementation Consortium (CPIC) analyzes the relationship between drugs and genes and stores relevant information in the PharmGKB database for clinical use. For example, a person with certain mutations responds poorly to an anticoagulant, resulting in an increased risk of heart attack.
How to use genetic research results in clinical practice is a time-consuming and labor-intensive task. However, combining genotype and phenotypic information can yield greater value. Most clinically relevant genetic mutations are identified by genome-wide association studies (GWAS). Researchers can now look backwards from medical records to determine what clinical manifestations are closely related to a genetic mutation.
Of course, the genome is only part of it, and other groups can also be a barometer of health.
Ultimately, patient involvement is required
As researchers strive to integrate data, the role of patients is beginning to emerge. For example, when we conduct research related to behavior, nutrition, exercise, smoking, and drinking, we need to rely on patient-reported data. Some wearable devices, such as smartphones and FitBits, are collecting exercise and heart rate data. Because of its ease of collection, this amount of data is constantly rising.
Therefore, everyone is a producer of big data. The data generated by ordinary people will far exceed the data accumulated in the clinic. We need to integrate data from these different sources for patient management. As people become more powerful in harnessing big data, patients will become the ultimate winner!
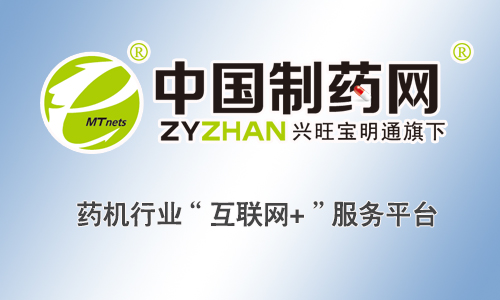
Seven major problems in the application of gene big data to clinical application need to be overcome
Massive data will bring unprecedented pressure on computational analysis and storage. It is estimated that genomics will soon exceed YouTube's data volume. But many researchers believe that today's big data is not rich enough and has no clinical value. "I don't know if 1 million is enough, but obviously we need more," said Marc Williams, dean of the Gesinger Genomics Medical Institute.
Application of variant data to clinical challenges and practices
Single nucleotide level mutation
At present, many research institutions mainly use exome sequencing, which can reduce the data analysis workload by nearly 100 times compared with the whole genome. However, there are still more than about 13,000 single nucleotide mutations identified. About 2% of these affect protein translation, and finding the disease-causing mutations is a daunting challenge.
For decades, researchers have put the single nucleotide mutation information they found into public resource databases, such as the dbSNP database. However, these mutational information often comes from the cellular level, animal models and even theoretical predictions, and is not sufficient for clinical diagnosis. In many cases, the level of evidence that these mutations are associated with the disease is very low.
Structural variation
Repeated or deleted genomic sequences that make clinical application more complicated. Existing sequencing techniques are difficult to detect structural variations. There are millions of variations between individuals within the genome-wide scope. Many of these mutations are located in non-coding regions, which do not encode proteins but have the effect of regulating gene activity and are still pathogenic. Since the scope and function of the non-coding region are difficult to define, even if the variation information can be obtained, clinical interpretation cannot be performed in a short period of time.
In response to these problems, people are working hard to solve them. For example, the National Human Genome Research Institute has established a Clinical Genome Resource Library, a disease-related mutation database with mutation information and supporting evidence to guide medical treatment. GenomicsEngland is advancing this by establishing a “clinical interpretation partnership†where doctors and researchers collaborate to build powerful disease-genotype association models.
The demand for large queues is obvious
Some "malignant" mutations are usually eliminated during evolution and are often rare, requiring large sample sizes for testing. Therefore, the establishment of a statistically significant model of mutation and disease-related correlation also requires a large number of patients.
Iceland's deCODE Genetics combines 150,000 genomic data (including 15,000 genome-wide sequences) with genealogy and medical history to infer the distribution of known genetic risk factors among the population, including breast cancer, diabetes and Alz Mutant mutations associated with the disease. As the company's CEO, Kári Stefánsson, said, "We have built 10,000 Icelandic databases with functional deletion mutations. We are investing a lot of energy to figure out how these gene deletions affect individuals."
The success of deCODE Genetics in this work is due to the genotype homogeneity of the Icelandic population, however, a broader genetic profile is required for Other projects. For example, the International Thousand Genome Project has catalogued some genetic diversity data, but most of the data are heavily biased towards Caucasians, making these data less useful for clinical research.
In addition, some of the questions are also derived from the reference genome. The first reference genome version is a patchwork of random breeder genes from several different races, but the latest version, GRCh38, integrates more information about the diversity of the human genome.
Generic data talent and computing power
Genomic or exome sequencing of large populations produces up to 40 PB (40 million GB) of data per year. In contrast, raw data storage is not the primary problem, and the bigger problem is the analysis of massive amounts of mutation data. Marylyn Ritchie, a genomics researcher at Penn State University, said, "The amount of computation is linear with the number of people. As variables and combinations increase, the amount of computation increases exponentially." If the increased data correlates with clinical symptoms or gene expression, Then the analysis will become more difficult. The processing of huge amounts of data from thousands of people may jeopardize many of the current statistical analysis tools. Ritchie said, "In the fields of meteorology, finance and astronomy, I have been exploring different types of data for a long time. I have communicated with people from Google and Facebook. Although our big data is different from them, we We should communicate more and apply their experience to our field."
However, unfortunately, many excellent programmers with big data mining experience have been attracted to Silicon Valley. Philip Bourne, deputy director of data science at the National Institutes of Health, believes that the scientific evaluation system is not suitable for these talents. Although some of these people really want to be scholars in the field of gene big data, they do not get a scholarship.
In addition to talent, data processing capabilities are another limiting factor. Gene big data often requires massively parallel computing using hundreds or thousands of large CPUs. To this end, many teams are turning to the "cloud" to store and analyze large amounts of data. TimHubbard, director of bioinformatics at Genomics England, said, "People are gradually getting this idea: applying algorithms to data." GenomicsEngland's cloud computing relies on government facilities, and its external access is strictly controlled. For other research institutions, the analysis of gene big data has gradually turned to commercial cloud systems, such as Amazon, Google and Ali's cloud services.
How to achieve data sharing and collaboration
In principle, cloud-based hosting encourages sharing and collaboration between databases. But highly sensitive clinical information and patient consent and privacy involve difficult ethical and legal issues.
In the European Union, different data processing rules between Member States hinder cooperation. Sharing data with non-EU countries relies on cumbersome mechanisms to establish data protection, and sharing data with private organizations requires a restrictive bilateral agreement. To help solve this problem, the Global Alliance for Genomics and Health has developed the Framework for Responsible Sharing of Genomic and Health-Related Data. The framework includes guidelines for privacy and informed consent, as well as the responsibilities and legal consequences of the organization that violates the rules.
Bartha Knoppers, bioethicist at McGill University in Canada and chairman of the Alliance's Regulatory and Ethical Working Group, said, “When signing a data transfer agreement, if the signatories agree to abide by the framework, they save a lot of work.†The framework allows Research organizations analyze shared genomic data while protecting privacy. Knoppers explained, “We hope to link these data to clinical data and medical records in the context of masking patients, otherwise we will not be able to achieve precision medicine.â€
In addition, the inclusion of genomics information in electronic medical archives has become increasingly important in many European countries. Hubbard said, "Our goal is to integrate it into the standard universal health insurance system." The UK's "100,000 genomics program" is at the forefront of this, but other countries are close behind. For example, Belgium recently announced a plan to explore medical genomics.
All of these countries benefit from this government-led public health insurance system. In the United States, the situation is more complicated. In addition to the public health insurance system, Medicare and Medicaid, the private health insurance system in the United States is very developed. Different medical insurance companies use different medical file systems, which makes the genome The integration of data has become difficult. In 2007, the National Institutes of Health funded the establishment of an electronic medical record and genomics network system (eMERGE) for big data integration and systems management analysis.
Clinical pharmacogenomics: from data to diagnosis
The integration of genomic data in medical records is mainly for doctors to provide reference for the diagnosis and treatment of diseases, one of which is pharmacogenomics. The Clinical Pharmacogenomics Implementation Consortium (CPIC) analyzes the relationship between drugs and genes and stores relevant information in the PharmGKB database for clinical use. For example, a person with certain mutations responds poorly to an anticoagulant, resulting in an increased risk of heart attack.
How to use genetic research results in clinical practice is a time-consuming and labor-intensive task. However, combining genotype and phenotypic information can yield greater value. Most clinically relevant genetic mutations are identified by genome-wide association studies (GWAS). Researchers can now look backwards from medical records to determine what clinical manifestations are closely related to a genetic mutation.
Of course, the genome is only part of it, and other groups can also be a barometer of health.
Ultimately, patient involvement is required
As researchers strive to integrate data, the role of patients is beginning to emerge. For example, when we conduct research related to behavior, nutrition, exercise, smoking, and drinking, we need to rely on patient-reported data. Some wearable devices, such as smartphones and FitBits, are collecting exercise and heart rate data. Because of its ease of collection, this amount of data is constantly rising.
Therefore, everyone is a producer of big data. The data generated by ordinary people will far exceed the data accumulated in the clinic. We need to integrate data from these different sources for patient management. As people become more powerful in harnessing big data, patients will become the ultimate winner!
Rfid Access Card,Rfid Access Control,Rfid Card Access Control,Access Control Rfid Reader
GRANDING TECHNOLOGY CO LTD , https://www.grandingsecurity.com